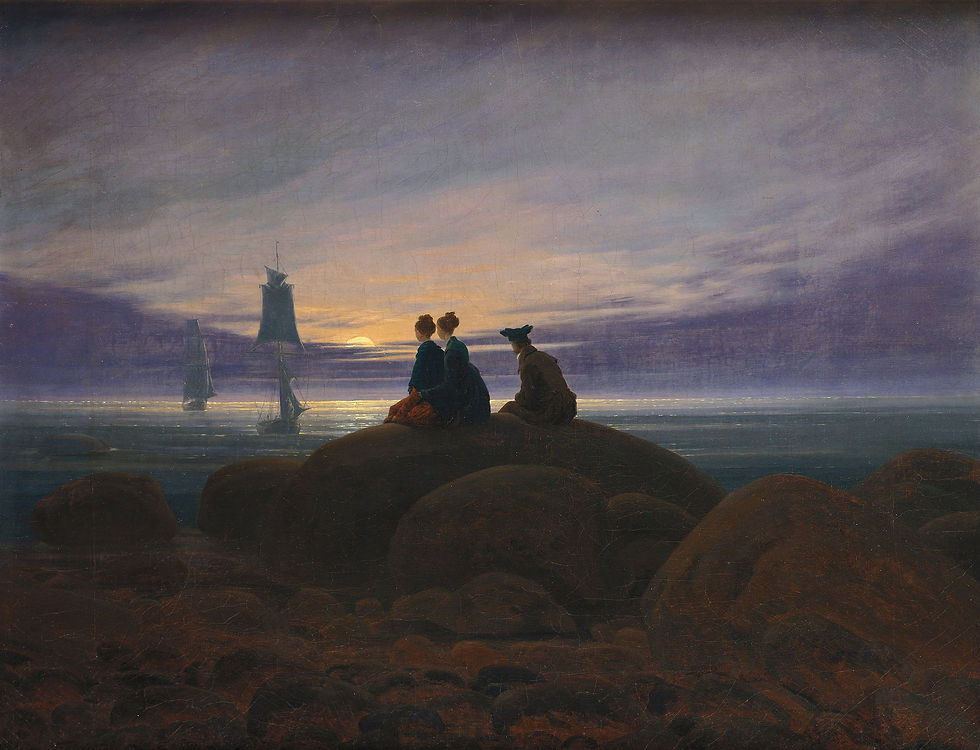
Causal Inference
Development of statistical methods in causal inference for observational and randomized controlled trial studies.
Causal inference deals with the identification and quantification of cause-effect relationships between phenomena. The understanding of the effects of causes (i.e., “What is the effect of A on B?”) and the causes of effects (i.e., “Is A a cause of B?”) is at the foundation of modern science (and our understanding of the world). Discovering, identifying, and quantifying causal relationships is a complex endeavor that requires sophisticated mathematical tools.
In my research, I focus on challenging real-world setups where rigorous statistical methods can provide answers to relevant questions that would be otherwise intractable. Examples include:
Personalized Treatment Regimes: People respond differently to treatments based on their unique characteristics. A one-size-fits-all approach is often suboptimal. My research focuses on heterogeneous treatment effects to develop tailored interventions.
Networks: Interventions often occur within interconnected networks, leading to network interference. This phenomenon causes treatment effects to "spill over" from treated units to their untreated connections. Understanding these dynamics is crucial for accurately assessing intervention impacts and designing effective policies that account for social and environmental interconnections.
Challenges in Randomized Control Trials: In some trials, units assigned to treatment do not receive it, causing broken randomizations. This occurs due to various factors like non-compliance or implementation issues. Studying these deviations provides valuable insights into causal pathways, revealing how interventions work in practice and informing better policy design.
My research in methodological and theoretical causal inference primarily centers on these challenging domains with a particular focus on the development of Bayesian causal inference methods. Below is a selection of my works in these domains.
Personalized Treatment Regimes: Discovery and inference of heterogeneous causal effects
Bargagli Stoffi, F. J., De Witte, K., Gnecco. G. Heterogeneous Causal Effects with Imperfect Compliance: a Bayesian Machine Learning Approach. Annals of Applied Statistics, 16 (3), 1986-2009, 2022.
[paper] Coverage: [R-bloggers post] [YoungStats post]
Zorzetto, D., Bargagli Stoffi, F.J., Canale, A., Dominici, F. Confounder-Dependent Bayesian Mixture Model: Characterizing Heterogeneity of Causal Effects in Air Pollution Epidemiology. Biometrics, 80(2), 2024.
[paper]
Bargagli Stoffi, F.J., Lee, K., Cadei, R., Dominici, F. Causal Rule Ensemble: Interpretable Discovery of Heterogeneous Subgroups. arXiv preprint arXiv:2009.09036.
[preprint]
2. Social Networks: Causal inference under network interference
Bargagli Stoffi, F.J., Tortù, C., Forastiere, L. Heterogeneous Treatment and Spillover Effects under Clustered Network Interference. Forthcoming at Annals of Applied Statistics.
[preprint]
Kim, R. C., Bargagli-Stoffi, F. J., Chen, K. L., Nethery, R. C. Towards Optimal Environmental Policies: Policy Learning under Arbitrary Bipartite Network Interference. arXiv preprint arXiv:2410.08362.
[preprint]
Chen, K.L., Bargagli Stoffi, F.J., Kim, R. C., Henneman, L., Nethery, R. C. Difference-in-Differences under Bipartite Network Interference: A Framework for Quasi-Experimental Assessment of the Effects of Environmental Policies on Health. arXiv preprint arXiv:2404.13442.
[preprint]
3. Challenges in Randomized Control Trials: Broken randomizations and causal pathways
Zorzetto, D., Canale, A., Mealli, F., Dominici, F., Bargagli Stoffi, F.J., Bayesian Nonparametrics for Principal Statification with Continuous Post-Treatment Variables arXiv preprint arXiv:2302.11656.
[preprint]
Bargagli Stoffi, F.J., Gnecco, G. Causal Tree with Instrumental Variable: An Extension of the Causal Tree Framework to Irregular Assignment Mechanisms. International Journal of Data Science and Analytics, 9, 315–337, 2020.
[paper]
Bargagli Stoffi, F.J., Gnecco, G. Estimating Heterogeneous Causal Effects in the Presence of Irregular Assignment Mechanisms. In IEEE 5th International Conference on Data Science and Advanced Analytics (DSAA) (pp. 1-10), 2018.
[paper]
Image: "Moonrise by the Sea", Caspar David Friedrich, 1822. Alte Nationalgalerie, Berlin, Germany.